Editor’s note: This post is part of a series showcasing Barcelona GSE master projects by students in the Class of 2018. The project is a required component of every master program.
Authors:
Michele Costa, Alessandro De Sanctis, Laurits Marschall and S. Hamed Mirsadeghi
Master’s Program:
Data Science
Paper Abstract:
The main goal of our Master Project is to predict intraday stock market movements using two different kinds of input features: financial indicators and sentiments from news and tweets. While the former are part of the common technical analysis of financial econometric models, the extracted sentiment of news articles and tweets from Twitter are also proven to correlate with stock markets movements. Our paper aims at contributing to the existing academic and professional knowledge in two main directions. First, we evaluate three different approaches to extract the sentiment from both social and mass media based on its forecasting power. Second, we deploy a battery of engineered features based on the sentiment, together with the financial indicators, in a machine learning model for a fine-grained minute-level forecasting exercise. In the end, two different classes of models are fitted to test the forecasting power of the combined input features. We estimated a classical ARIMA-model, and an XGBoost-model as machine learning algorithm. We collected data on the companies Apple, JPMorgan Chase, Exxon Mobil, and Boeing.
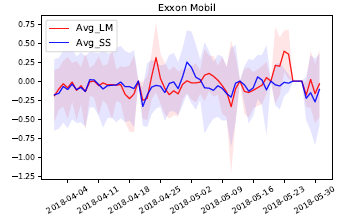
More about the Data Science Program at the Barcelona Graduate School of Economics