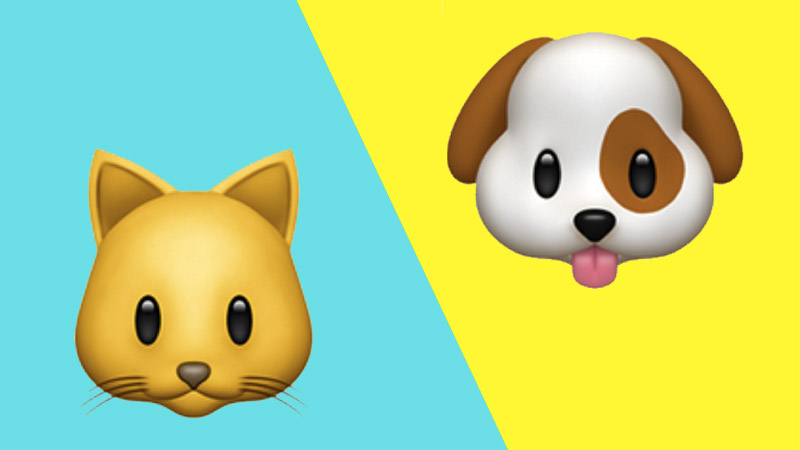
Is artificial intelligence (AI) trustworthy? If, like me, you have recently been gobsmacked by the Netflix documentary Coded Bias, then you were probably quick to answer that question with a definite “no”. The show documents the efforts of a group of researchers headed by Joy Buolamwini, that aims to inform the public about the dangers of AI.
One particular place where AI has already wreaked havoc is automated decision making. While automation is intended to liberate decision making processes of human biases and judgment error, it all too often simply encodes these flaws, which at times leads to systematic discrimination of individuals. In the eyes of Cathy O’Neil, another researcher appearing on Coded Bias, this is even more problematic than discrimation through human decision makers because “You cannot appeal to [algorithms]. They do not listen. Nor do they bend.” What Cathy is referring to here is the fact that individuals who are at the mercy of automated decision making systems usually lack the necessary means to challenge the outcome that the system has determined for them.
In my recent post on Towards Data Science, I look at a novel algorithmic solution to this problem. The post is based primarily on a paper by Joshi et al. (2019) in which the authors develop a simple, but ingenious idea: instead of concerning ourselves with interpretability of black-box decision making systems (DMS), how about just providing individuals with actionable recourse to revise undesirable outcomes? Suppose for example that you have been rejected from your dream job, because an automated DMS has decided that you do not meet the shortlisting criteria for the position. Instead of receiving a standard rejection email, would it not be more helpful to be provided with a tailored set of actions you can take in order to be more successful on your next attempt?
The methodology proposed by Joshi et al. (2019) and termed REVISE is an attempt to put this idea into practice. For my post I chose a more light-hearted topic than job rejections to illustrate the approach. In particular, I demonstrate how REVISE can be used to provide individual recourse to Kitty 🐱, a young cat that identifies as a dog. Based on information about her long tail and short overall height, a linear classifier has decided to label Kitty as a cat along with all the other cats that share similar attributes (Figure below). REVISE sends Kitty on the shortest possible route to being classified as a dog 🐶 . She just needs to grow a few inches and fold up her tail (Figure below).
The following summary should give you some flavour of how the algorithm works:
- Initialise x, that is the attributes that will be revised recursively. Kitty’s original attributes seem like a reasonable place to start.
- Through gradient descent recursively revise x until g(x*)=🐶. At this point the descent terminates since for these revised attributes the classifier labels Kitty as a dog.
- Return x*-x, that is the individual recourse for Kitty.
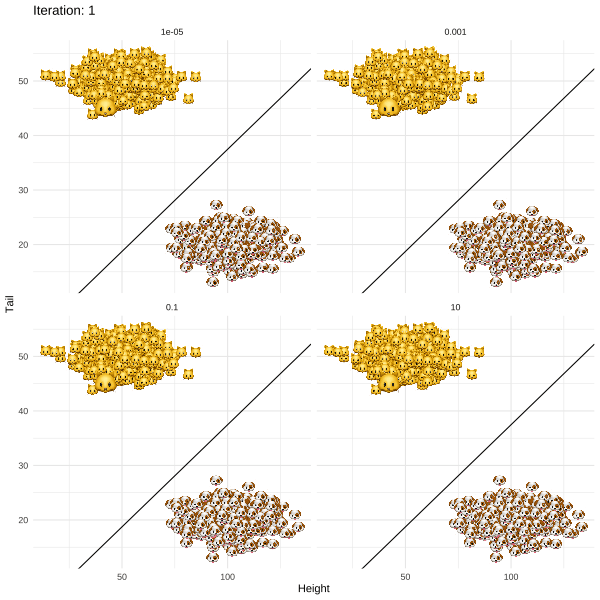
This illustrative example is of course a bit silly and should not detract from the fact that the potential real-world use cases of the algorithm are serious and reach many domains. The work by Joshi et al. adds to a growing body of literature that aims to make AI more trustworthy and transparent. This will be decisive in applications of AI to domains like Economics, Finance and Public Policy, where decision makers and individuals rightfully insist on model interpretability and explainability.
Further reading
The article was featured on TDS’ Editor’s Picks and has been added to their Model Interpretability column. This link takes you straight to the publication. Readers with an appetite for technical details around the implementation of stochastic gradient descent and the REVISE algorithm in R may also want to have a look at the original publication on my personal blog.
Connect with the author
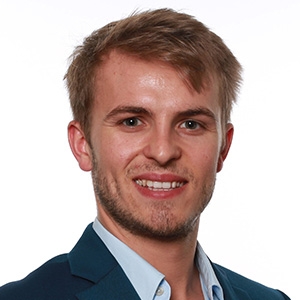
Following his first Master’s at Barcelona GSE (Finance Program), Patrick Altmeyer worked as an economist for the Bank of England for two years. He is currently finishing up the Master’s in Data Science at Barcelona GSE.
Upon graduation Patrick will remain in academia to pursue a PhD in Trustworthy Artificial Intelligence at Delft University of Technology.