A team of Barcelona GSE Data Science students from the Class of 2017 will compete in the final round of the Data Science Game in Paris at the end of September.
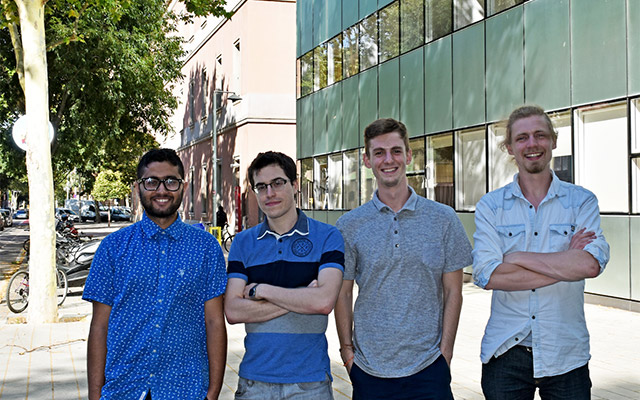
A team of Barcelona GSE Data Science students from the Class of 2017 will compete in the final round of the Data Science Game in Paris at the end of September.
Among 400 international teams from 220 universities that participated in the first round, the BGSE team is among the 20 teams who have qualified for the final. The team is called “Just Peanuts” and its members are Roger Garriga, Javier Mas, Saurav Poudel, and Jonas Paul Westermann.
In the following interview, they talk about the Data Science Game and their expectations for the final.
What is the Data Science Game?
The Data Science Game is an annual Data Science competition for university students organized by ENSAE (Paris). Teams of up to four people can participate and represent their university. There is a free-for-all qualification round online and the top 20 teams are invited to the Finale in Paris.
Why did you decide to participate?
During the course we already took part in one data science challenge as part of the Computational Machine Learning course. That was quite fun and we have been generally wanting to take part in Kaggle-like challenges throughout the year. On top of that, we of course need to represent the Barcelona GSE and put the word out about our amazing Master’s.
Can you explain the task your team had to perform in the first round of the game?
The challenge for the online qualification round was related to predicting user’s music preferences. Data was provided by Deezer, a Music streaming service based in France. The training dataset consisted of 7+ million rows each pertaining to one user-song interaction describing weather the user listened to the song (for longer that 30 seconds) or not and whether the song was suggested to the user by the streaming service as well as further variables relating to the song/user.
How/by whom was the first round judged/scored?
The online round was hosted on Kaggle, a common website for these kinds of data science prediction challenges. Scoring was done according to the ROC AUC metric (reciever operator characteristic Area under the curve).
Was it difficult to combine participating in the game with your courses and assignments in the master program?
As we started really investing time into the challenge only quite late (about two weeks before the end) we spent a lot of time during the final days. The last 120 hours before submission were probably entirely spent on the challenge which definitely cut into our normal working schedules. Especially the last weekend before the deadline was very intense and spent mostly sitting shirtless at the table of a very overheated apartment living off frozen pizza and chips.
What specifically from the master’s helped you succeed in the game?
Part of the final model we used and what also made the first miles in terms of achieving a good score was a library recommended by one of the PhD students who also give lectures in our course. But also beyond that, we used all kinds of background knowledge and experience gained from the course. A constant scheme during the challenge were problems with difference in distribution and construction of the training and testing datasets. This gave inaccurately high cross-validation results and made it difficult to assess the quality of predictions.
Another issue was simply the size of the data that meant training and parameter tuning were extremely time consuming and we needed to expand our infrastructure beyond our own laptops. For both of those problems we’ve talked about possible solutions during the Master’s and applied combinations thereof.
What will you have to do for the final round? Can you tell us about your strategy or will that give too much information to the other teams?
The final round will be a two-day hackathon-like data science challenge on-site in Paris. No information has been shared with us on details of the challenge but we are thinking it might be something related to sound processing to continue the theme from part one.
How can we follow your progress in the competition?
We will surely be writing an update after the Paris trip and probably also give some social media updates during the event.