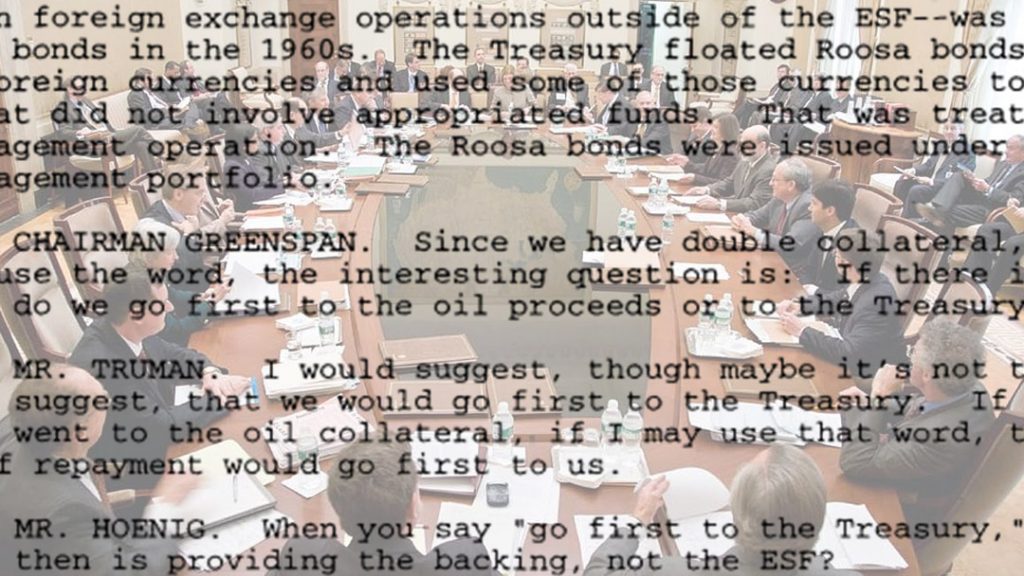
Editor’s note: This post is part of a series showcasing BSE master projects. The project is a required component of all Master’s programs at the Barcelona School of Economics.
Abstract
In this study, we propose an approach for the extraction of a low-dimensional signal from a collection of text documents ordered over time. The proposed framework foresees the application of Latent Dirichlet Allocation (LDA) for obtaining a meaningful representation of documents as a mixture over a set of topics. Such representations can then be modeled via a Dynamic Linear Model (DLM) as noisy realisations of a limited number of latent factors that evolve with time. We apply this approach to Federal Open Market Committee (FOMC) speech transcripts for the period of Greenspan presidency. This study serves as exploratory research for the investigation into how unstructured text data can be incorporated into economic modeling. In particular, our findings point at the fact that a meaningful state-of-the-world signal can be extracted from expert’s language, and pave the way for further exploration into the building of macroeconomic forecasting models, and in general into the usage of variation in language for learning about latent economic conditions.
Key findings
In our paper, we develop a sequential approach for the extraction of a low-dimensional signal from a collection of documents ordered over time. We apply this framework to the US Fed’s FOMC speech transcripts for the period 08-1986 to 01-2006. We retrieve estimates for a single latent factor, that seem to track fairly well a specific set of topics connected with risk, uncertainty, and expectations. Finally, we find a remarkable correspondence between this factor and the Economic Policy Uncertainty Indices for United States.
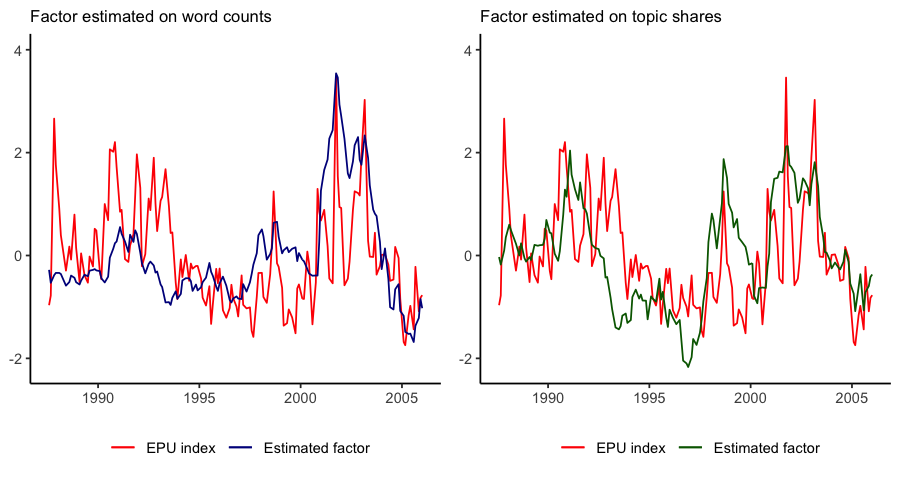
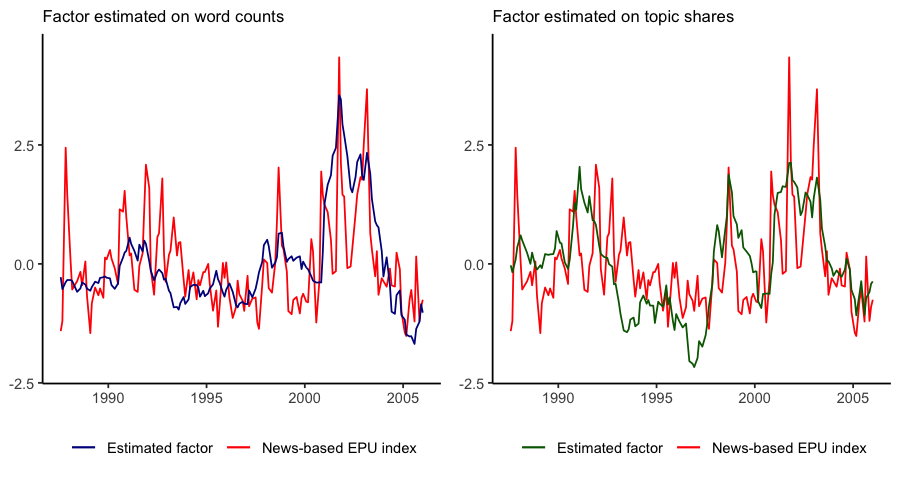